Overview
Dashboards provide real-time insights into the performance and health of systems and applications within an organization. They allow users to visually analyze data, track key performance indicators (KPIs), and monitor trends efficiently. With dashboards, teams can identify anomalies, prioritize issues, proactively detect problems, diagnose root causes, and ensure that reliability goals are met. Empower your teams to make informed decisions, optimize system operations, and drive business success by providing a centralized and user-friendly interface for monitoring and analyzing critical metrics and performance indicators.
Get started
To create a dashboard:
- Click +New Dashboard on the Dashboard List page or New Dashboard from the navigation menu.
- Enter a dashboard name and choose a layout option.
- Dashboards
- A grid-based layout, which can include a variety of objects such as images, graphs, and logs. They are commonly used as status boards or storytelling views which update in real time, and can represent fixed points in the past. They have a maximum width of 12 grid squares and also work well for debugging.
- Timeboards
- Automatic layouts that represent a single point in time—either fixed or real-time—across the entire dashboard. They are commonly used for troubleshooting, correlation, and general data exploration.
- Screenboards
- Dashboards with free-form layouts which can include a variety of objects such as images, graphs, and logs. They are commonly used as status boards or storytelling views that update in real time or represent fixed points in the past.
See the following resources:
Refresh rate
The refresh rate of a private dashboard depends on the time frame you are viewing. The shorter the time frame is, the more frequently the data is refreshed. Publicly shared dashboards refresh every thirty seconds, regardless of the selected time frame.
Time frame | Refresh rate |
---|
1 minute | 10 seconds |
2 minutes | 10 seconds |
5 minutes | 10 seconds |
10 minutes | 10 seconds |
30 minutes | 20 seconds |
1 hour | 20 seconds |
3 hours | 1 minute |
4 hours | 1 minute |
1 day | 3 minutes |
2 days | 10 minutes |
1 week | 1 hour |
1 month | 1 hour |
3 months | 1 hour |
6 months | 1 hour |
1 year | 1 hour |
View dashboards on mobile devices
View your dashboards in a mobile-friendly format with the Datadog Mobile App, available on the Apple App Store and Google Play Store. The Mobile App comes equipped with mobile home screen widgets that allow you to monitor service health and infrastructure without opening the mobile app.
Note: To set up or edit a dashboard, you must log in to the Datadog browser UI. For more information on installing the app, see the Datadog Mobile App documentation.
Further Reading
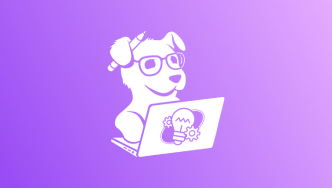
Try Creating Graph Widgets in the Datadog Learning Center
Explore timeseries, query value, top list, table, distribution, and pie chart widgets. Learn how to configure the widgets and develop an understanding of when each widget type should be utilized.
ENROLL NOW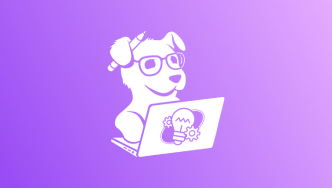
Try Creating Table, List, SLO, and Architecture Widgets in the Datadog Learning Center
Explore table, list, SLO, and architecture widgets. Learn how to track the metrics and performance of a web application and discover how to present important data.
ENROLL NOWAdditional helpful documentation, links, and articles: